Will AI cause a large-scale industrial accident?
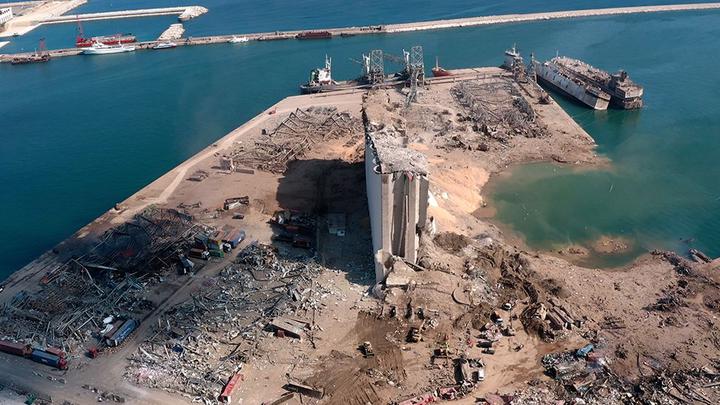
The tragedy that occurred in Beirut this month is a chilling reminder of how short-term thinking and organizational blind spots endanger all our lives. Here, sadly, it appears the mistakes were human. Large amounts of dangerous materials were improperly stored and then, apparently, forgotten.
This got me thinking: what could happen if an AI system was managing the shipping and logistics of one or more major ports? Could a sophisticated but improperly specified algorithm, which is difficult for its operators to audit or interpret, let a similar accident happen?
The fears of AI being misused in an industrial setting are not new, and a growing body of work has studied this problem. However, much of that work explores either artificial general intelligence, which still does not exist, or robots. An algorithm controlling an industrial robot1 needs safety protocols and a physically grounded understanding of the world, so it can understand what nearby humans are doing and how they will react to the robot’s actions.
But here I’m talking about a more ephemeral, and in many ways more practical, application of AI. Even without robots, AIs and automation still play a huge role—and be a huge danger—within a complex system like the global supply chain.
AI Supply Chains
Computerized scheduling is already central to the global supply chain. I had a job unloading trucks in college, and even in 2000, the managers would often be surprised by what the computers had decided to ship when we’d open the trucks. One time the computers got it wrong and we had to scramble to find a place to stash hundreds of children’s bikes!
Modern logistics and shipping is dominated by algorithms. Look at any Amazon Fulfillment center and all the robotic bookcases whizzing by the workers. But as machine learning becomes ever more practical, it seems an obvious tool to squeeze ever more efficiency out of the supply chain. Yet not all machine learning methods are interpretable, and what can go wrong if a so-called black box method starts making decisions?
Now imagine a scenario like those extra children’s bikes, only it’s potentially explosive materials, and it’s happening in a high-speed environment where people may not even realize the material’s danger?
And given the scale of industrial accidents—and the interconnected complex system of the global supply chain—the dangers may be far higher than those posed by self-driving cars.
Network effects
It seems straightforward to flag conditions on goods for safety and proper handling. Indeed, the computer will likely be better than people at checking for issues. But what about more complex situations?
What if a mixture of two or more materials, none dangerous alone, but hazardous together, get stored in proximity? This combinatorial problem could also, in principle, be mitigated by computer checks. But what if there are competing firms, renting shipping space nearby, each using their own algorithms to ship and schedule materials?
When you throw interactions between potentially discordant systems into the mix, all hell could break loose. Multiple, competing black boxes, or even a combination of black box and interpretable AI, could lead to extremely unpredictable and dangerous scenarios.
Now we can see the interest and urgency in studying XAI.
Winter or crash?
Neural Network research went through two significant setback periods, known as AI winters. People often use the AI winters of the 1970s and 1990s to predict the future of AI. However, those past AI winters were about research interest: Problems arose that made neural networks appear untenable, and researchers shifted to alternatives. The 2010s have obliterated those concerns, cementing neural networks' place in practice. AI is too important to go away now. So it seems lessons from the previous AI winters are unlikely to apply.
If an AI system were to cause a large-scale industrial accident, it’s plausible that another AI winter could occur. However, now that AI is so successful in practice, an AI winter here would be quite different than researchers losing interest. Instead, it may be more akin to a stock market crash, with “buyers” fleeing and the market collapsing. The shine goes off because an accident has been made clear how dangerous AI can be, in a more visceral way than Skynet could ever be. Companies could pledge to slow or stop their research and development of AI systems. Or perhaps government regulation, for better or worse, comes in when legislators realize the broader safety implications of what has been wrought.
Fighting the last war
Lastly, it’s worth pointing out short-termism in my thoughts here.
The Beirut explosion just happened. I am focused on that same scenario: thousands of tons of improperly stored explosives-in-waiting. Someone who really works on these problems must be up all night, wracking their brain trying to foresee other possibilities. What if an AI diverts sewage treatment incorrectly? What if harmful manufacturing additives are accidentally added to containers for medicine?
What are the dangerous, unknown and truly unanticipated situations?
-
Or a self-driving car. ↩︎